Status for: Water Reservoir (MOD28)
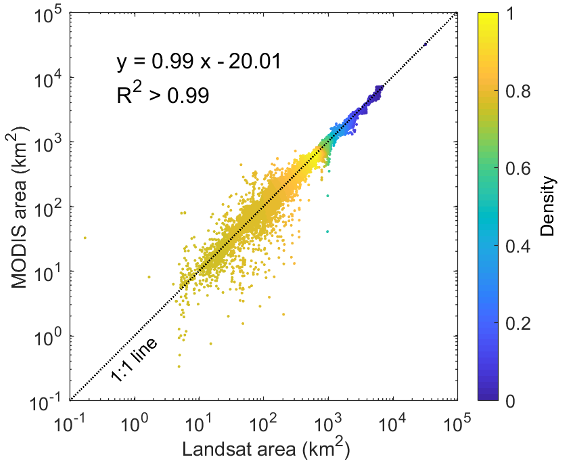
Figure 1. Comparison of monthly area estimations between Landsat and
MODIS from February 2000 to December 2018 for the 164 reservoirs.
Note that the x-axis and y-axis use a logarithmic scale, and there are a total of 37228 (227x164) pairs.
Stage 1 Validation has been achieved for the MODIS MxD28 Collection 6.1 Global Water Reservoir product.
The MxD28 Global Water Reservoir product-including time series of surface area, elevation, storage, evaporation rate, and volumetric evaporation - has been validated to Stage 1 using Landsat measurements and in situ observations.
At the global scale, long-term in situ reservoir area records are still lacking. Therefore, we compared the MODIS area values with Landsat based results (at a finer spatial resolution of 30m) for purposes of area validation (Figure 1). The Landsat monthly reservoir area values for all of the 164 reservoirs were collected from Global Reservoir Surface Area Dataset (GRSAD) between 2000 and 2018 [Zhao and Gao 2018].
For the elevation and storage validations, we collected in situ daily observations for twelve Indian reservoirs (Ukai, Matatila, Rana Pratap Sagar, Gandhi Sagar, Ban Sagar, Bargi, Hirakud, Jayakwadi, Sriram Sagar, Nagarjuna Sagar, Yeleru, and Tungabhadra) from the Indian Central Water Commission (http://cwc.gov.in/) between 2000 and 2019. We used the Indian reservoirs for validation purposes because they experience large dynamics that can better evaluate the efficiency of our algorithm
The validation results of the 8-day MODIS elevation and storage products are shown in Figure 2 and Figure 3, respectively. Overall, the elevation estimations from MODIS agree well with the in situ data (Figure 2), with an average R2 value of 0.87, an average RMSE value of 2.22 m, and an average NRMSE value of 12.28%. The biases (e.g., overestimation for Tungabhadra and underestimation for Yeleru) are caused by a combination of mixed pixels of reservoir edge, parameterization of the enhancement algorithm, and the mismatch of MODIS water areas with Landsat water areas, which were used to derive Area-Elevation relationships for Global Reservoir Bathymetry Dataset [Li et al. 2020]. With regard to the storage validations (Figure 3), they have similar patterns with those of the elevation results because they were both derived from area time series. Validations against in situ data show an average R2 value of 0.88, an average RMSE value of 0.47 km3, and an average NRMSE value of 13.20%.
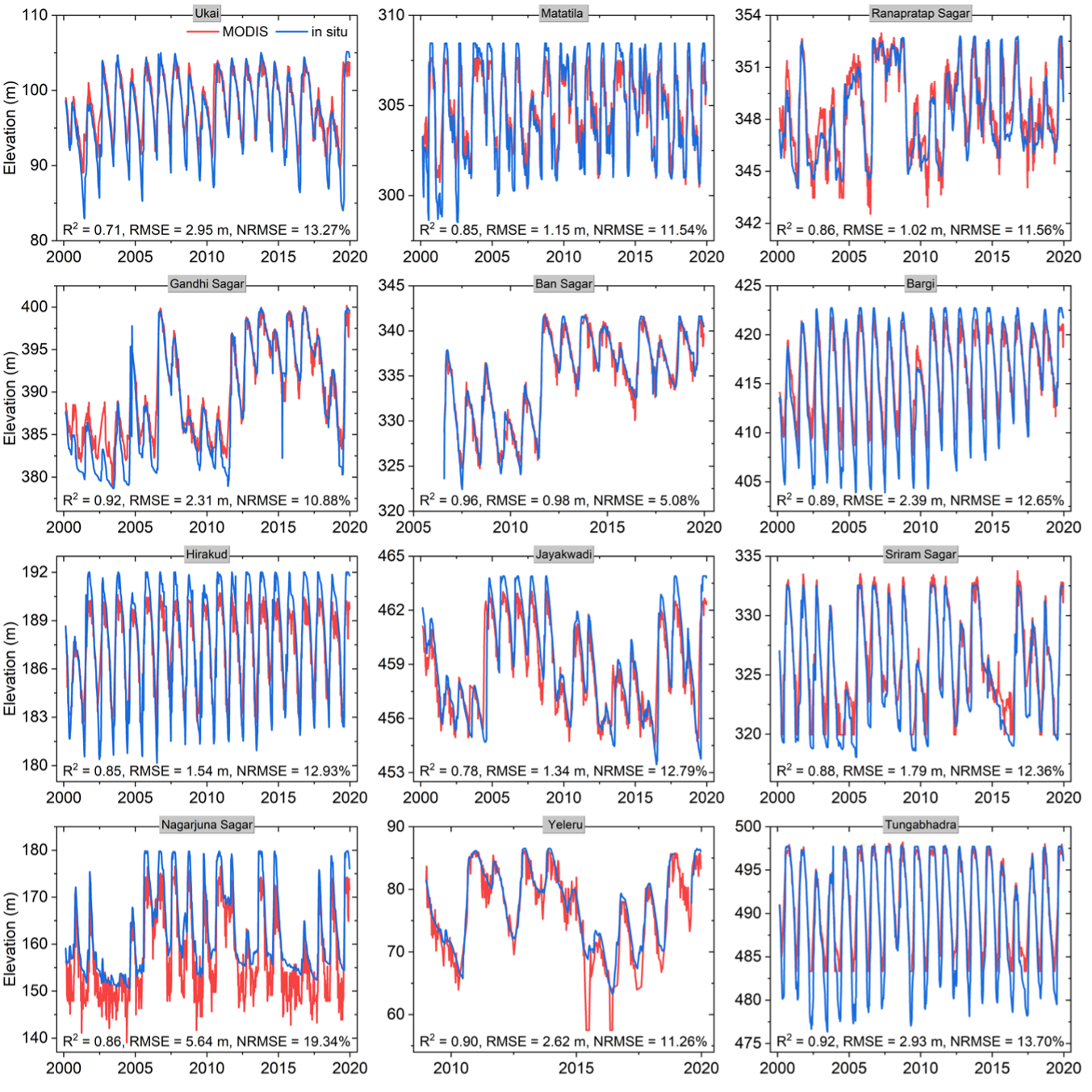
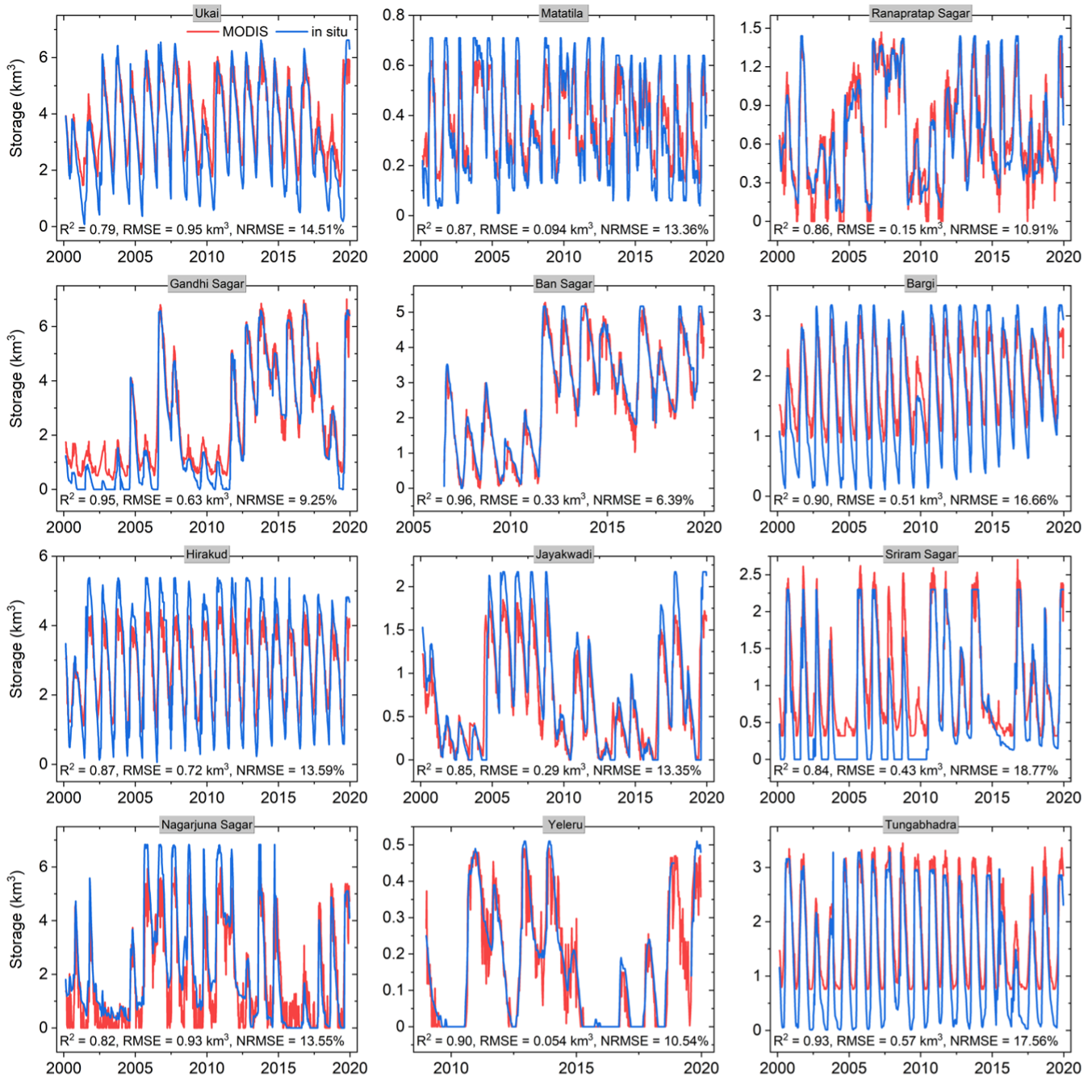
Figure 2. Validation of MODIS 8-day elevation products for twelve
Indian reservoirs from 2000 to 2019.
Click on graphic to see larger version
Figure 3. Validation of MODIS 8-day storage products for twelve
Indian reservoirs from 2000 to 2019.
Click on graphic to see larger version
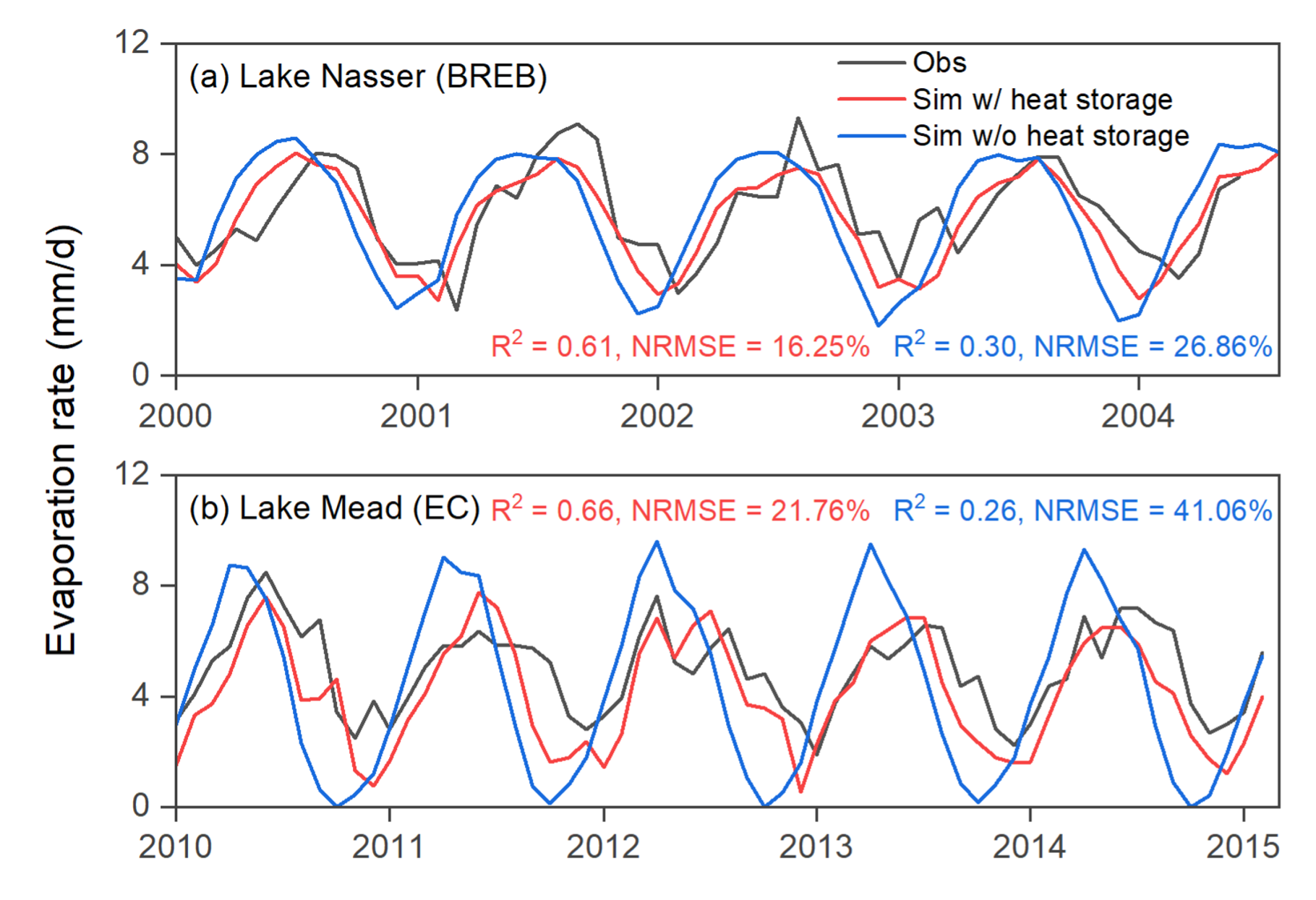
Figure 4. Validation of the evaporation rates for (a) Lake Nasser and
(b) Lake Mead using Bowen ratio energy budget (BREB) estimations and
eddy covariance (EC) measurements.
Next, we validated the monthly elevation and storage products over these twelve Indian reservoirs. The daily in situ elevation and storage values were averaged at a monthly step, which were then compared to the monthly MODIS products. The validation results show similar patterns as those of the 8-day products, but higher accuracies (figures not shown). This is because the monthly reservoir area values were generated from the composited results of three or four 8-day reservoir areas from MxD28C2, and the composition process greatly reduced the adverse effects of cloud contamination at the 8-day time step. The MODIS based elevations show good consistency with the in situ measured data, with an average R2 value of 0.90, an average RMSE value of 1.99 m, and an average NRMSE value of 11.33%. Regarding the storage validations, the results are consistent with those of the elevations-with an average R2 value of 0.91, an average RMSE value of 0.43 km3, and an average NRMSE value of 11.91%.
The evaporation rate results were validated over two locations-Lake Nasser in Africa, and Lake Mead in North America-where in situ observations are available. The in situ evaporation rate values for Lake Nasser and Lake Mead were collected using Bowen ratio energy budget (BREB) estimations and eddy covariance (EC) measurements, respectively. As shown in Figure 4, the MODIS evaporation rate products have good overall agreement with values obtained via observation (with R2 values of 0.61, and 0.66 and NRMSE values of 16.25% and 21.76%). Compared to the results which were calculated using the regular Penman equation (without heat storage), our MODIS evaporation rate estimates have shown great improvement in terms of both annual peak values and seasonal variations. For Lake Nasser, the R2 value increases from 0.30 to 0.61, with the NRMSE decreasing from 26.86% to 16.25%. A greater improvement is observed for Lake Mead in terms of both R2 (from 0.26 to 0.66) and NRMSE (from 41.06% to 21.76%). These results suggest that better evaporation rate estimates are achieved when considering heat storage within the algorithm. It should be noted that this evaporation rate algorithm has been validated at more locations in Zhao et al. (2020).
Product version: Collection 6.1
Title: NASA's MODIS/VIIRS Global Water Reservoir Product suite from moderate resolution remote sensing data |
Author: Yao Li; Gang Zhao; Deep Shah; Maosheng Zhao; Sarkar Sudipta; Sadashiva Devadiga; Bingjie Zhao; Shuai Zhang; and Huilin Gao |
Source: Remote Sensing, 2021 |
View Abstract and Access Publication |
Title: A high-resolution bathymetry dataset for global reservoirs using multi-source satellite imagery and altimetry |
Author: Li, Y., Gao, H., Zhao, G., Tseng, K.H. |
Source: Remote Sensing of Environment, 2020, 244, 111831 |
View Abstract and Access Publication |
Title: Estimating lake temperature profile and evaporation losses by leveraging MODIS LST data |
Author: Gang Zhao; Huilin Gao; Ximing Cai |
Source: Remote Sensing of Environment, 2020, 251, 112104 |
View Abstract and Access Publication |
Title: Estimating reservoir evaporation losses for the United States: Fusing remote sensing and modeling approaches |
Author: Zhao, G.,Gao, H. |
Source: Remote Sensing of Environment, 2019, 226, 109-124 |
View Abstract and Access Publication |
Title: Deriving High-Resolution Reservoir Bathymetry from ICESat-2 Prototype Photon-Counting Lidar and Landsat Imagery |
Author: Li, Y., Gao, H., Jasinski, M.F., Zhang, S., Stoll, J.D. |
Source: IEEE Transactions on Geoscience and Remote Sensing, 2019, 57, 10(7883-7893) |
View Abstract and Access Publication |
Title: Automatic correction of contaminated images for assessment of reservoir surface area dynamics |
Author: Zhao, G.,Gao, H. |
Source: Geophysical Research Letters, 2018, 45, 12 |
View Abstract and Access Publication |
Title: Monitoring reservoir storage in South Asia from multisatellite remote sensing |
Author: Shuai, Z., Gao, H., Naz, B.S. |
Source: Water Resources Research, 2014, 50, 11 |
View Abstract and Access Publication |
Title: Global monitoring of large reservoir storage from satellite remote sensing |
Author: Gao, H, Birkett, C., Lettenmaier, D.P. |
Source: Water Resources Research, 2012, 48, 9 |
View Abstract and Access Publication |